The most effective actions,
every day, in every store
The performance of a retail network relies on the precision of its action plans and their speed of implementation.
But it is difficult to be sure that what's needed is being done every day in every store.
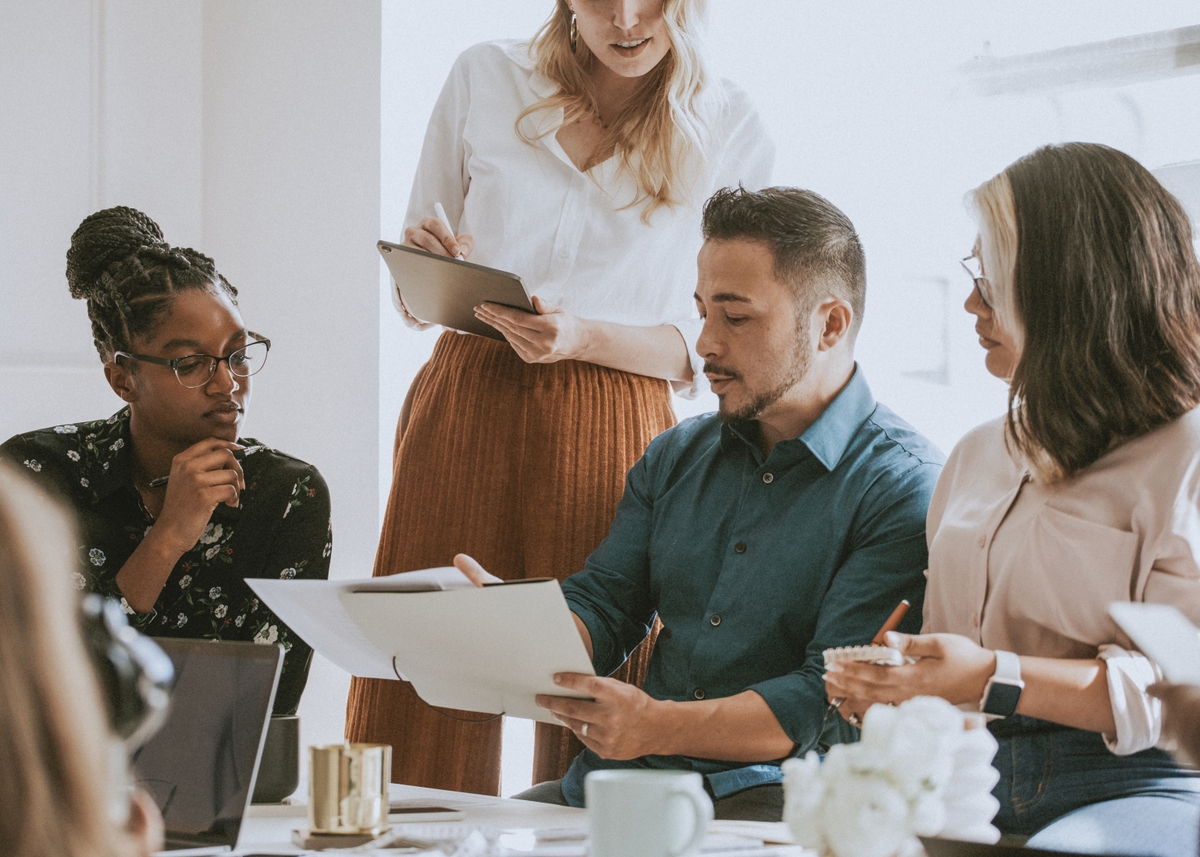
Making the right diagnoses and determining the appropriate actions for each point of sale is beyond the reach of headquarters analysts.
And the availability and analytical skills of field managers cannot guarantee that the right actions are taken on time.
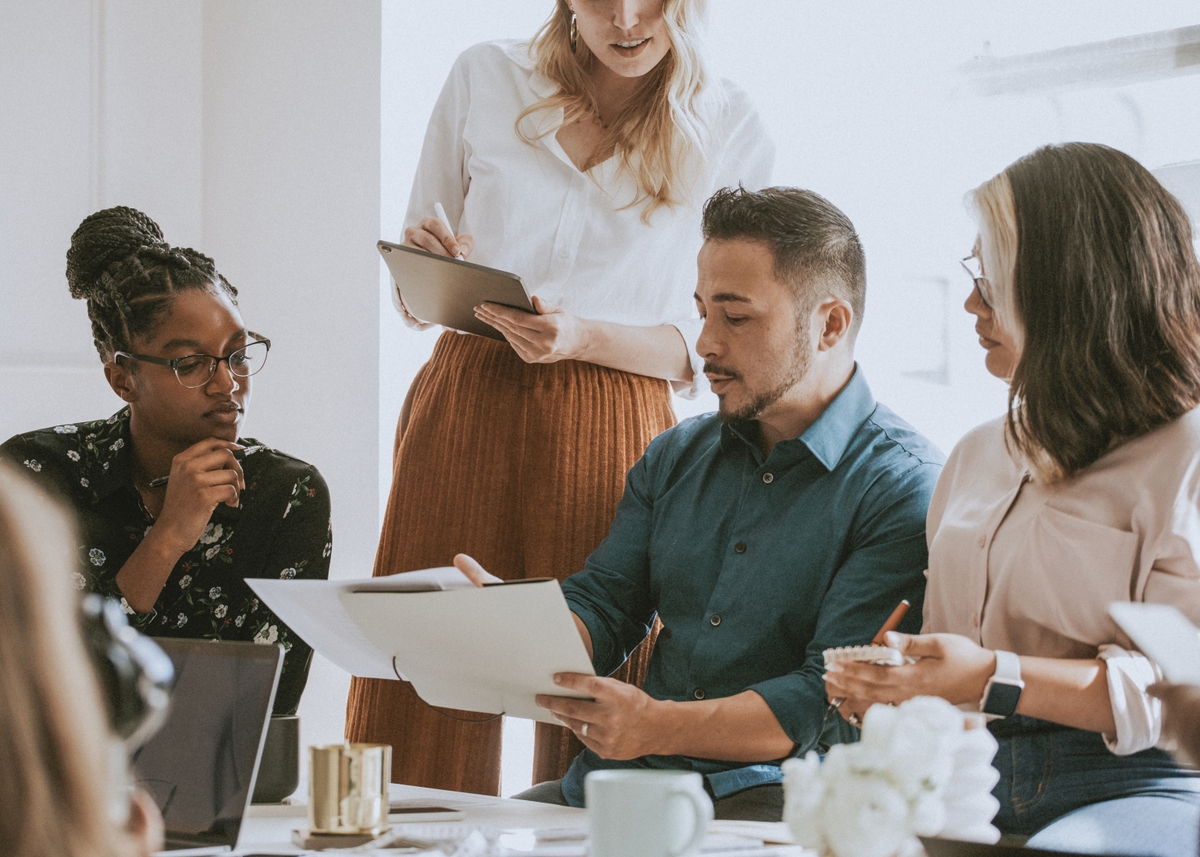
Opportunities of the week
Our algorithms analyze the sales of each store and benchmark them with other stores of the chain that have similar customer profiles. Performance gaps are opportunities to be seized.
The most valuable opportunities are sent to the managers in charge so that they can choose the actions to be taken for the week (visual merchandizing, inventory, training, challenge...).
As a result, the chosen actions are perfectly suited to the context and potential of each store, and taken early enough in the week to have an impact by the week-end.
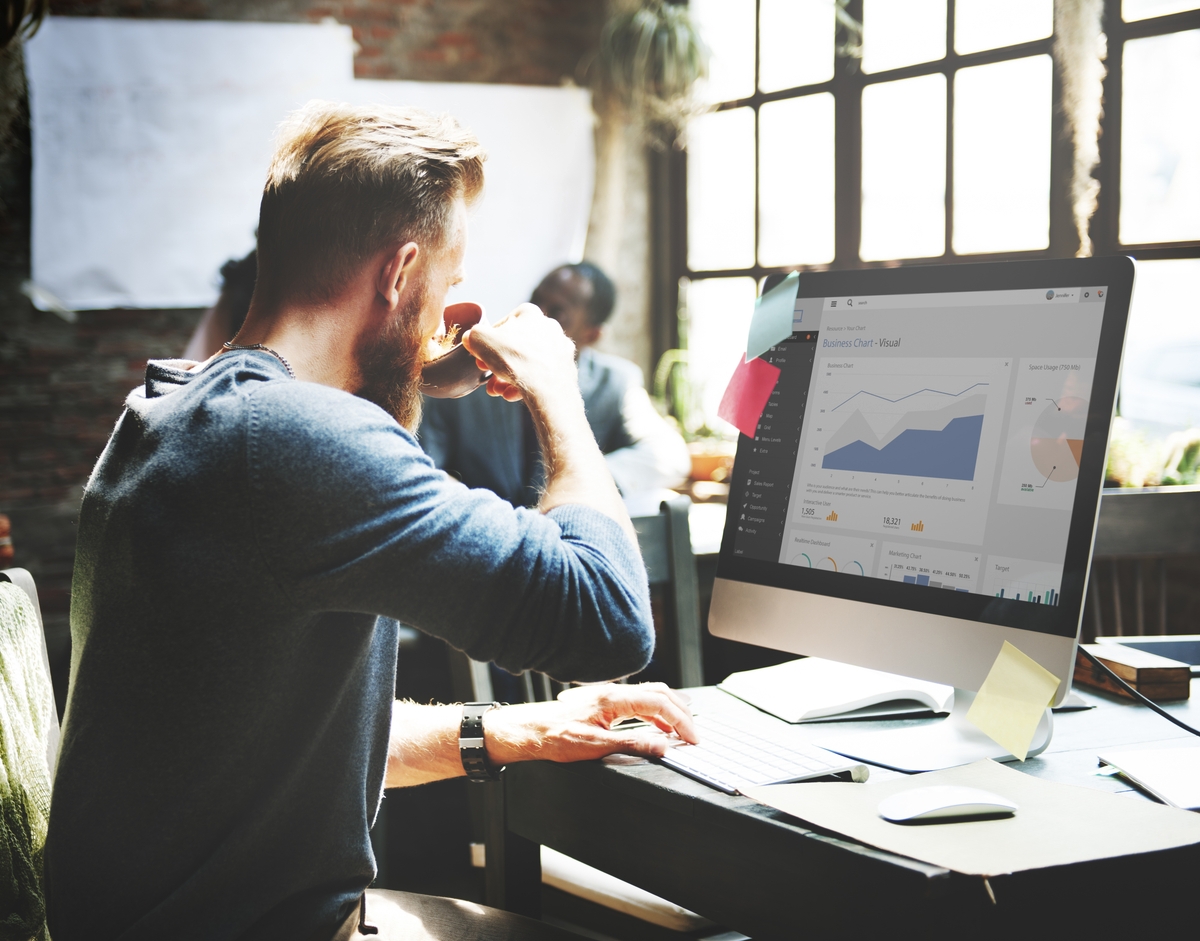
Synthesizing actions taken on the field is laborious, and rigorous analysis of their impact on performance is complex.
So you rarely have visibility over what is being done and what is actually working.
And even more when actions are taken continuously throughout the year, as they should.
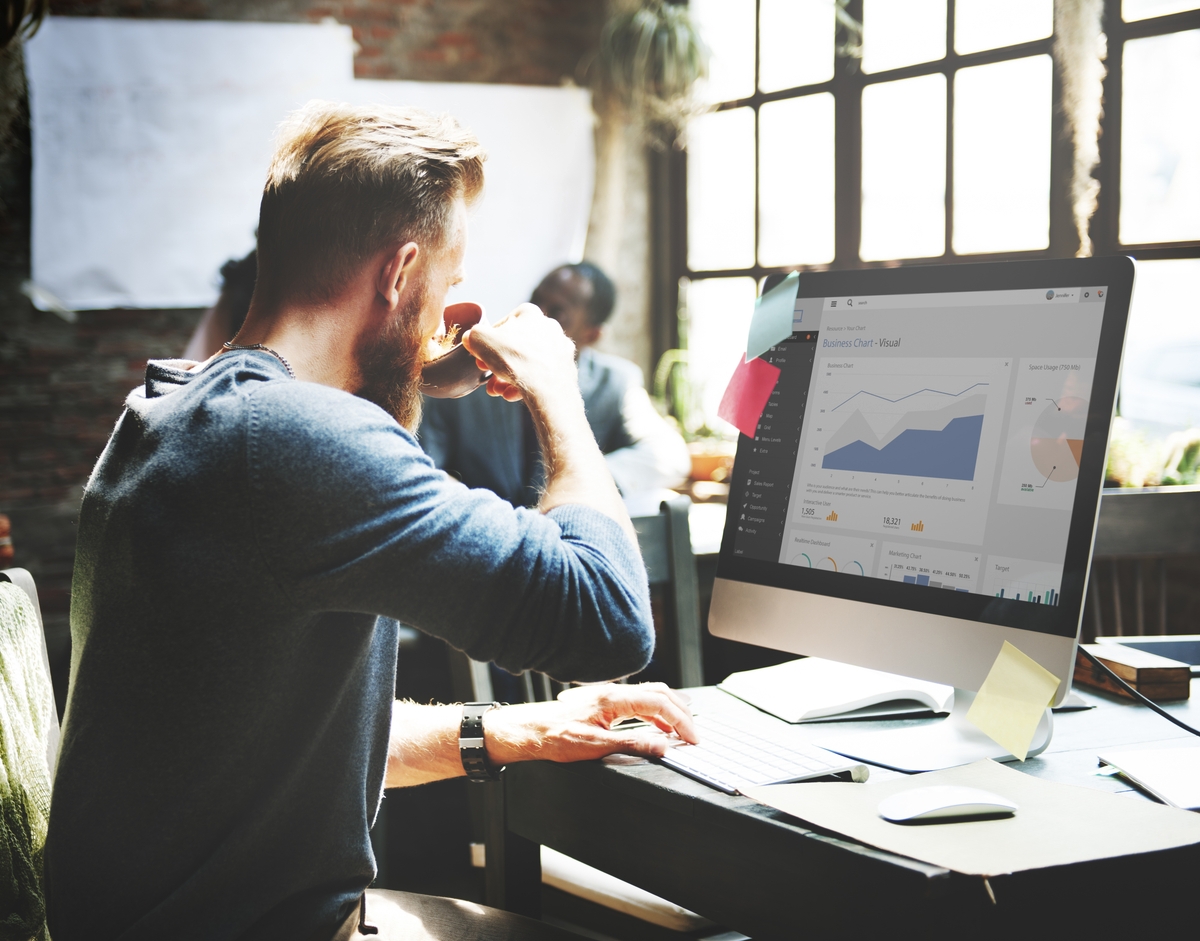
.png)
Tracking the impact of your actions
Our solution records the actions taken and their progress status, relieving managers of the need for laborious reporting.
Regional management has an overview of the actions in progress in each point of sales within its scope.
It is possible to identify the actions taken most frequently across the network, to identify needs for support from the head office.
After a few weeks of use, users can discover the most impactful actions according to the context and targeted products.
.png)

In any network, there is great diversity between the various points of sales due to the diversity of the clientele and contextual factors.
Therefore we frequently end up comparing stores that have nothing in common. This makes performance benchmarks useless and lacking credibility in the eyes of operational staff, whereas it could be an extraordinary steering and motivational tool.

Your comparable stores
Our algorithms group together stores which are visited by similar customer profiles with similar behaviors.
These stores therefore have similar sales potential at the time of the benchmark. Therefore it makes sense to compare their performances.
This 100% data-driven clustering outperforms fixed segmentations by taking into account seasonal changes in shopping behavior, which do not happen at the same time in all regions.
Higher retail performance, thanks to data
from the first week of usage
every week